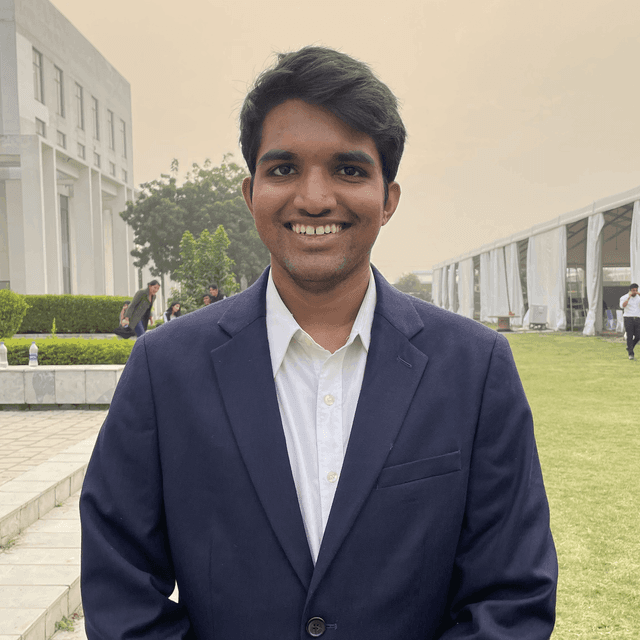
Mann Acharya
TensorFlow Certified ML Engineer, Engineer @ ZeroThreat.ai
About
Hello! I'm Mann. I break business and life challenges into optimization problems and implement iterative solutions to match or surpass human-level performance.
My expertise lies in search engines, computer vision, edge deployment, and recommender systems. I have built end-to-end AI products encompassing the entire software development life cycle (Frontend, Backend, AI, and MLOps).
I do:
- AI Research
- Open Source
- AI Consulting
- Community building
Reach please reach out to reach out using my socials!

News
May 2024:
Student Engineer at Develop4Good
February 2024:
Joined ZeroThreat.ai as Foundational Machine Learning Engineer
February 2024:
Joined Lyfelynk as Techincal Project Manager
Work
Ashoka - Develop For Good
Student Engineer●May 2024 - Aug 2024
LyfeLynk
Technical Project Manager (Part-time)●Feb 2024 - Present
ZeroThreat.ai
Foundational AI Engineer (Full-time)●Feb 2024 - Present
Samsung Display Noida
Computer Vision Engineer (Consultant)●Feb 2024 - Mar 2024
AtheroPoint
AI Research Engineer (Part-time)●Oct 2023 - Feb 2024
Radixweb
Computer Vision Intern (Part-time)●May 2023 - Oct 2023
Azimuth
Machine Learning Engineer (On-Contract)●May 2023 - Aug 2023
DXFactor
Machine Learning Intern (Full-time)●Dec 2022 - Jan 2023
Bennett Artificial Intelligence Society
President (Volunteer)●Aug 2023 - Present
Indian Blockchain Fraternity
Technical Head (Volunteer)●Sep 2022 - Jul 2023
Projects


Publication


Machine Learning Models for Enhancing Time Series Demand Forecasting in Business Intelligence
Released In: June 2024
We analyzed the efficiency of four machine learning models—ANN, LSTM, Decision Tree, and Linear Regression—in demand forecasting using a textile company's sales data. ANN provided the most accurate forecasts with the lowest data loss, while LSTM was slightly less accurate. These findings highlight the importance of advanced models in optimizing inventory management and supply chain efficiency.

Strategic Applications of Deep Belief Networks in Anomaly Detection and Generative Modeling for Business Intelligence
Released In: June 2024
This study compares Temporal-DBN, Variational-DBN, and Sparse-DBN models for anomaly detection in business intelligence, finding Temporal-DBN significantly outperforms with a 97.67% accuracy, indicating its potential to enhance data-driven decision-making in diverse business fields.